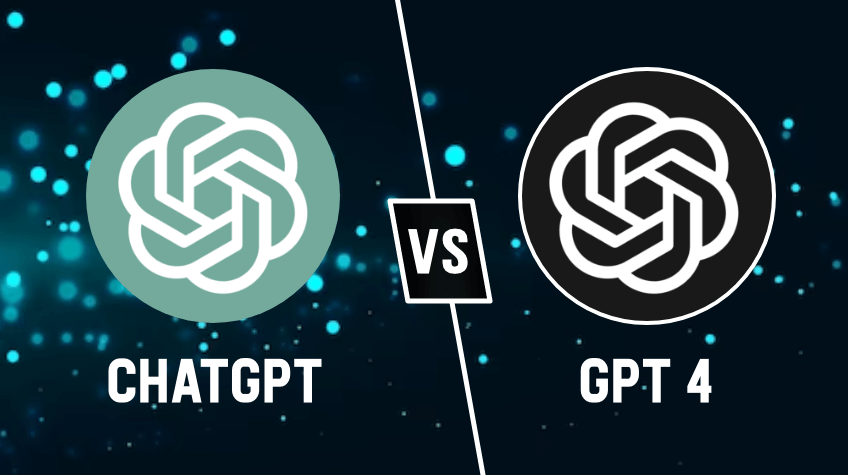
Introduction
The field of artificial intelligence has been evolving at an astonishing pace, and one of the most exciting developments in recent years has been the progression of generative language models. Among these, OpenAI’s GPT series has consistently stood out as a benchmark for natural language understanding and generation. With the release of GPT-4.0, we find ourselves at the forefront of AI innovation, marking a significant step forward from its predecessor, GPT-3.5. In this comprehensive article, we will delve into the differences between these two iterations, exploring their advancements, capabilities, and potential implications.
GPT 4.0 Model Architecture
The fundamental architecture of GPT-4.0 remains rooted in the transformer architecture, much like its predecessor, GPT-3.5. However, it is important to note that GPT-4.0 incorporates several enhancements in its architecture, resulting in improved performance and capabilities.
Model Size and Parameters
One of the most noticeable differences is the increase in model size and parameters. GPT-4.0 is significantly larger, with billions of parameters, dwarfing the already impressive GPT-3.5. This expanded size allows GPT-4.0 to capture a broader spectrum of language nuances and context, making it even more proficient in understanding and generating human-like text.
Advanced Architecture
GPT-4.0 also incorporates advanced architectural features, including novel attention mechanisms and deeper layers. These enhancements enable the model to handle more complex linguistic tasks, such as reasoning, summarization, and translation, with greater accuracy and coherence. The model’s architecture has been fine-tuned to minimize common issues such as repetitive responses and nonsensical outputs, which were more prevalent in GPT-3.5.
Training Data
Another significant difference between GPT-3.5 and GPT-4.0 lies in the training data. Both models were trained on vast corpora of text from the internet, but GPT-4.0 benefits from more recent data, which means it has a better understanding of current events, trends, and evolving language patterns. This improved training data has a direct impact on the model’s ability to generate relevant and up-to-date content, making it a more valuable tool for various applications.
Language Capabilities
The core objective of generative language models like GPT-3.5 and GPT-4.0 is to understand and generate human-like text. However, the enhancements in GPT-4.0 led to several notable improvements in its language capabilities.
Contextual Understanding
GPT-4.0 demonstrates a deeper understanding of context, allowing it to maintain more coherent and contextually relevant conversations. This advancement is particularly noticeable in longer interactions, where GPT-3.5 might occasionally lose track of the conversation’s context.
Multimodal Understanding
While GPT-3.5 primarily focuses on text-based inputs and outputs, GPT-4.0 extends its capabilities into the realm of multimodal understanding. This means it can process and generate text-based responses for a wider range of inputs, including images, audio, and video. This ability opens up exciting possibilities for applications in areas like content generation, multimedia captioning, and more immersive user experiences.
Fine-tuning and Customization
Both GPT-3.5 and GPT-4.0 support fine-tuning, allowing developers and organizations to adapt the models for specific tasks or domains. However, GPT-4.0’s enhanced architecture and training data make it more adaptable and easier to fine-tune. This results in improved performance when tailoring the model for niche applications, such as chatbots, virtual assistants, and content generation.
Ethical Considerations
As AI models become more powerful, ethical considerations become increasingly important. OpenAI has made efforts to address ethical concerns with both GPT-3.5 and GPT-4.0, including guidelines on responsible AI usage and content moderation. However, GPT-4.0 includes additional safeguards and fine-tuning options to mitigate issues related to biased or harmful outputs, a challenge that was more pronounced in GPT-3.5.
Applications
The differences between GPT-3.5 and GPT-4.0 have profound implications for a wide range of applications. Here, we explore some of the key areas where GPT-4.0 shines:
Natural Language Processing
In the field of Natural Language Processing (NLP), GPT-4.0 raises the bar for tasks such as text generation, summarization, sentiment analysis, and language translation. Its improved contextual understanding and larger model size result in more accurate and coherent outputs.
Virtual Assistants and Chatbots
Virtual assistants and chatbots powered by GPT-4.0 offer more engaging and context-aware interactions. The model’s ability to comprehend nuanced queries and provide relevant responses makes it an invaluable tool for customer support, e-commerce, and general user assistance.
Content Generation
GPT-4.0 is a game-changer in content generation, enabling the automatic creation of high-quality articles, product descriptions, marketing copy, and more. Its enhanced understanding of context and multimodal capabilities make it an ideal tool for content creators.
Healthcare and Research
In healthcare and research, GPT-4.0’s advanced capabilities can assist in tasks like medical literature analysis, drug discovery, and patient data interpretation. Its fine-tuning options make it adaptable to specific healthcare domains.
Creative Writing and Storytelling
For creative writers and storytellers, GPT-4.0 can serve as a source of inspiration and assistance. It can generate plot ideas, and character descriptions, and even help with editing and revision
Challenges and Concerns
Despite the remarkable advancements in GPT-4.0, it is not without its challenges and concerns:
Computational Resources
The increased model size of GPT-4.0 demands substantial computational resources for training and inference. This could limit its accessibility for smaller organizations and developers with limited computing resources.
Ethical Considerations
As AI models become more capable, the responsibility of ethical AI usage becomes paramount. GPT-4.0’s potential to generate biased or harmful content requires careful oversight and moderation.
Data Privacy
The use of vast amounts of training data raises concerns about data privacy and potential information leakage. It is crucial to implement robust data protection measures when using GPT-4.0 for sensitive applications.
Future Prospects
GPT-4.0 represents a significant milestone in the field of generative language models, but it is not the end of the road. The pace of AI research and development continues to accelerate, and we can expect even more powerful and capable models in the future. As GPT-4.0 paves the way for these advancements, it is essential to stay vigilant and consider the ethical and societal implications of AI technology.
Conclusion
In the ongoing journey of AI advancement, GPT-4.0 emerges as a remarkable successor to GPT-3.5, pushing the boundaries of natural language understanding and generation. With its larger model size, improved architecture, and enhanced capabilities, GPT-4.0 is poised to transform a wide range of industries and applications, from NLP and virtual assistants to content generation and healthcare.
However, it is important to acknowledge that along with its potential, GPT-4.0 also brings challenges and ethical considerations that need to be addressed responsibly. As we continue to explore the capabilities of this model and its successors, we must remain vigilant and proactive in ensuring that AI technology benefits society while minimizing potential risks.
GPT-4.0’s evolution highlights the dynamic nature of the AI landscape, where breakthroughs in architecture, training data, and ethical guidelines pave the way for more versatile and reliable AI systems. It also underscores the importance of ongoing research, development, and collaboration within the AI community.
Looking ahead, here are some key areas to watch as GPT-4.0 and similar models continue to evolve:
- Increased Accessibility: Efforts to make advanced AI models more accessible to a wider range of users, including small businesses and individuals with limited resources, will likely continue. This could involve the development of user-friendly interfaces and cloud-based solutions that reduce the computational burden.
- Ethical AI: Ethical considerations remain a central concern. AI developers and organizations will need to prioritize responsible AI usage, transparency, and accountability. Ongoing efforts to reduce biases and mitigate harmful outputs will be crucial.
- Regulation and Oversight: Governments and regulatory bodies are becoming more involved in AI oversight. The introduction of regulations and standards for AI model development and deployment will play a significant role in shaping the industry’s future.
- Multimodal AI: As GPT-4.0 introduces multimodal capabilities, we can anticipate further advancements in models that can understand and generate text, images, audio, and video seamlessly. This will lead to more immersive and interactive applications across various domains.
- Customization and Fine-Tuning: AI models like GPT-4.0 will continue to be adapted and fine-tuned for specific industries and applications. This will drive innovation in areas such as healthcare, finance, education, and entertainment.
- Research and Development: Ongoing research in AI, particularly in natural language processing and machine learning, will drive the development of even more sophisticated models. Future iterations may have even larger model sizes and capabilities we can only imagine today.
Aspect | GPT-3.5 | GPT-4.0 |
---|---|---|
Model Size | Large model with millions of parameters | Significantly larger model with billions of parameters |
Language Understanding | Good contextual comprehension | Enhanced language understanding and contextual awareness |
Multimodal Capabilities | Primarily text-based inputs and outputs | Introduction of multimodal understanding for images, audio, and video |
Fine-Tuning | Supports fine-tuning for specific tasks | Improved adaptability and ease of fine-tuning |
Error Reduction | Some issues with ambiguous or erroneous responses | Fine-tuned to minimize common issues, leading to fewer errors |
Content Generation | Proficient at generating content but may require revisions | Maybe less accessible to smaller organizations or those with limited computing resources |
Ethical Considerations | Ethical guidelines and content moderation | Additional safeguards and options for reducing biased or harmful outputs |
Training Data | Trained on a substantial corpus of internet text | Benefits from more recent training data for up-to-date language understanding |
Computational Resources | Requires substantial computational resources | Demands significant computational power for training and inference |
Accessibility | Availability to developers with varying resources | May be less accessible to smaller organizations or those with limited computing resources |
In conclusion, the transition from GPT-3.5 to GPT-4.0 marks a significant leap forward in the field of generative language models. GPT-4.0’s enhanced architecture, training data, and capabilities promise to reshape industries and unlock new possibilities for human-machine interactions. However, with great power comes great responsibility, and ethical considerations, data privacy, and regulation will continue to play critical roles in shaping the future of AI.
As we navigate this evolving landscape, it is crucial to strike a balance between innovation and ethical considerations, ensuring that AI technology remains a force for positive change in society. With ongoing collaboration, research, and responsible development, we can harness the potential of models like GPT-4.0 to drive progress while mitigating potential risks. The journey of AI advancement is far from over, and the possibilities are limitless.